Ocean's "big data": fusion of satellite data for better sea views
KNOXVILLE, TN, December 21, 2024 /24-7PressRelease/ -- Scientists have unveiled a groundbreaking method (CSAC) to harmonize satellite ocean color data across various satellites, which paved the way for the generation of reliable, global-scale, long-term, bio-optical properties of the upper ocean. Such "big data" is the base to assess the status and climate-related dynamics of the marine ecosystems. The CSAC (Cross-Satellite Atmospheric Correction) system ensures consistent remote sensing reflectance (Rrs) products across different satellite ocean color missions, a crucial step for expanding spatial coverage and extending the temporal reach via the fusion of various satellite ocean color measurements.
Since the late 1990s, ocean color satellites have revolutionized the monitoring of the upper ocean, providing critical insights into the spatial distribution and temporal changes of many important properties, such as clarity and the "load" of phytoplankton. However, discrepancies among satellite ocean color missions have persisted due to differences in sensor design and atmospheric correction algorithms, complicating efforts to merge data from different missions. Addressing these discrepancies is essential for generating comprehensive, long-term datasets needed to monitor climate impacts on the oceans.
The State Key Laboratory of Marine Environmental Science at Xiamen University, in collaboration with the National Satellite Ocean Application Service, has announced a major study (DOI: 10.34133/remotesensing.0302) published on November 7, 2024, in Journal of Remote Sensing. The research introduces CSAC (Cross-Satellite Atmospheric Correction), an innovative system that aligns top-of-atmosphere reflectance data from various satellites to match the highest-quality Rrs compiled by MODIS (Moderate Resolution Imaging Spectroradiometer)-Aqua. This method effectively resolves persistent data inconsistencies, setting the stage for robust, multi-decadal ocean monitoring crucial for understanding climate change.
CSAC marks a significant leap forward in satellite ocean color data processing. Unlike conventional atmospheric correction approaches, which require sensor-specific algorithms, CSAC employs artificial intelligence to process top-of-atmosphere reflectance data from multiple satellites to a standard Rrs database uniformly. At the heart of this advancement is a meticulously curated, highest-quality dataset derived from 20+ years of MODIS-Aqua observations. This dataset serves as a reliable reference, enabling CSAC to standardize data from sensors like SeaWiFS (Sea-Viewing Wide Field-of-View Sensor) and MERIS (MEdium Resolution Imaging Spectrometer). In testing, CSAC reduced discrepancies in Rrs across wavelengths, cutting MAPD (mean absolute percentage differences) by up to 50% compared to traditional methods. This underscores CSAC's capability to deliver consistent and accurate ocean color records, indispensable for tracking marine ecosystems and assessing global climate trends.
Dr. Zhongping Lee, one of the study's lead researchers, emphasized, "The CSAC system represents a significant advancement in satellite ocean color remote sensing. By harnessing decades of the highest-quality MODIS-Aqua data and sophisticated machine-learning techniques, we have resolved critical inconsistencies in Rrs among different satellites. This not only improves data reliability but also empowers the scientific community to create accurate, long-term records of ocean bio-optical properties, essential for climate studies." He highlighted the importance of consistent ocean monitoring to deepen our understanding of climate change.
The implications of CSAC are far-reaching for oceanographic research and satellite ocean color remote sensing. By ensuring consistency in satellite-derived bio-optical data, scientists can now produce reliable, long-term data products from multiple satellite missions. Such datasets are vital for observing shifts in ocean ecosystems, examining the ocean's role in the carbon cycle, and evaluating climate change impacts. Furthermore, CSAC's AI-based approach sets a benchmark for future satellite data processing, highlighting that this processing is reaching a new era: transitioning from radiative-transfer-based approaches to data-based systems.
References
DOI
10.34133/remotesensing.0302
Original Source URL
https://spj.science.org/doi/10.34133/remotesensing.0302
Funding information
The financial support from the National Natural Science Foundation of China (#42430107 and #42250710150), the National Key Research and Development Program of China (2022YFC3104903), Fujian Satellite Data Development, Co., Ltd., and Fujian Haisi Digital Technology Co., Ltd. We thank NASA for the distribution of SeaWiFS and MODIS ocean color products. Comments and suggestions from 2 anonymous reviewers are greatly appreciated.
About Journal of Remote Sensing
The Journal of Remote Sensing, an online-only Open Access journal published in association with AIR-CAS, promotes the theory, science, and technology of remote sensing, as well as interdisciplinary research within earth and information science.
Chuanlink Innovations, where revolutionary ideas meet their true potential. Our name, rooted in the essence of transmission and connection, reflects our commitment to fostering innovation and facilitating the journey of ideas from inception to realization.
Related Link:
http://chuanlink-innovations.com
Read the full story here: https://www.24-7pressrelease.com/press-release/517333/oceans-big-data-fusion-of-satellite-data-for-better-sea-views
Press Release Distributed by 24-7Pressrelease.com
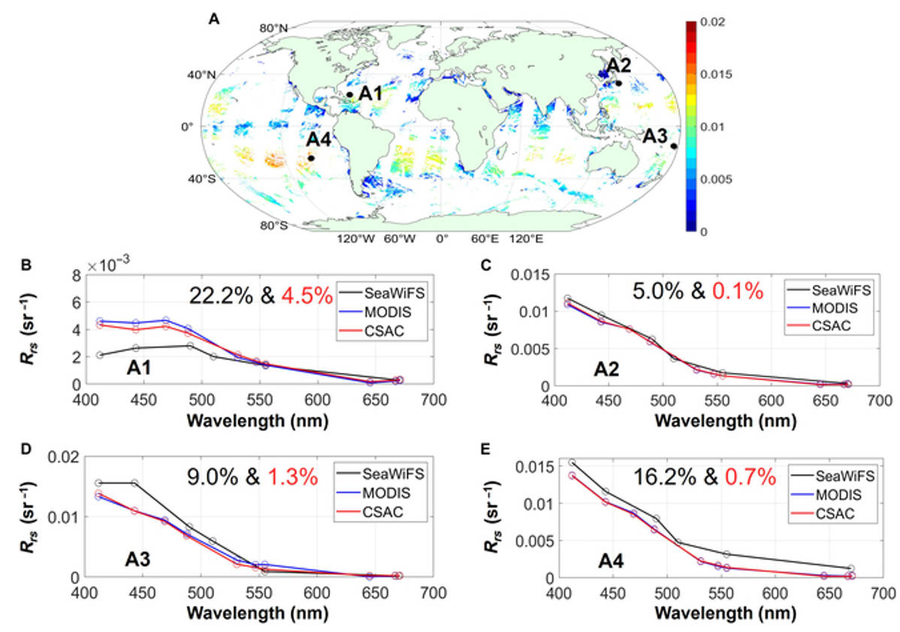
Ocean's "big data": fusion of satellite data for better sea views
24-7 Press Release Newswire